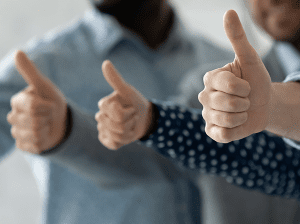
“Can I trust this data?”
In the dawning age of artificial intelligence (AI), this question becomes increasingly critical for individuals and organizations. Data reliability is the cornerstone of an organization’s data-driven decision-making. A recent survey from Precisely identified data-driven decision-making as the primary goal of 77% of data initiatives, yet only 46% of organizations have high or very high trust in the data that supports their decisions, according to the study.
A report from the World Economic Forum highlights the importance of data reliability in realizing the potential of AI. While 90% of public and private CEOs believe AI is essential to counteracting climate change, 75% of executives don’t have a high level of trust in the reliability of the data that powers their crucial data projects. Ensuring the success of future data-driven initiatives starts with trustworthy data, and proving that data is trustworthy begins by defining what data reliability is, and determining how to achieve it.
What Is Data Reliability?
Data reliability is the determination that the data is accurate, complete, consistent, and free of errors. Ensuring the reliability of data is a component of an organization’s data integrity efforts, which extend beyond the data itself to the infrastructure and processes related to the data:
- Physical integrity governs the procedures for safely storing and retrieving data from IT systems. It protects against outages and other external threats to data’s reliability.
- Logical integrity confirms that the data makes sense in various contexts. The logic of data can be compromised by human error or flaws in system design. Logical integrity has four aspects:
- Domain integrity relates to the acceptable range of values, such as integers, text, or date.
- Entity integrity prevents duplication by applying primary keys that uniquely identify records in a relational database table.
- Referential integrity implements rules and procedures that maintain consistency between two database tables.
- User-defined integrity attempts to identify errors that the other integrity checks miss by applying the organization’s own internal rules and limitations on the data.
Data reliability serves as the first step in creating robust data-driven decision-making processes. The quality of decisions is affected by the incompleteness of the data, data inaccuracies, and biases introduced by the lack of standardization of data formats, inconsistent data definitions, and improper data collection methods. Having confidence in the reliability of your data allows decision-makers to gather the information they need and respond quickly to changing industry and market conditions.
Why Is Data Reliability Important?
One way to measure the importance of data reliability is by considering the characteristics of unreliable data:
- Inaccurate data is flat-out wrong and misleading.
- Outdated data is no longer accurate and equally misleading.
- Incomplete data is missing values or lacks specific attributes, such as a customer record without contact information.
- Duplicate data can skew analyses and waste resources.
- Inconsistent data exists in different forms or formats within the organization.
- Irrelevant data doesn’t add value in the context of the current analysis.
- Unstructured data lacks a context that allows it to be analyzed accurately, such as plain text vs. text in a defined database field.
- Non-compliant data causes problems for regulated industries such healthcare and finance and can lead to legal and financial penalties.
Conversely, reliable data improves the quality of business decisions, contributes to the company’s operational efficiency, boosts customer satisfaction levels, makes financial management more accurate, and facilitates regulatory compliance. Other benefits of data reliability to an organization are more effective marketing, lower operating costs, more accurate forecasting, enhanced scalability, and more meaningful and useful data integrations.
The most important advantage firms gain from greater data reliability may be the trust that they build with employees, partners, and customers. If trust is the foundation of business relationships, data reliability is the pathway to establishing strong, long-lasting ties and positive interactions with parties and stakeholders inside and outside the company.
How to Measure Data Reliability
The first step in measuring data reliability is to determine the most appropriate metrics for the specific type of data and application, or “dimension.” Some metrics for data reliability are intrinsic, or independent of a particular use case, such as the total number of coding errors in a database. Others are extrinsic, meaning they’re tied directly to a specific task or context, such as a web page’s average load time.
Intrinsic metrics encompass data accuracy, completeness, consistency, freshness, and privacy and security:
- Accuracy is measured by how well the data describes or represents the real-world situation to which it pertains. This includes whether the data possesses the attributes described in the data model, and whether the model’s predictions about events and circumstances prove to be true.
- Completeness relates to both the data itself and the data models that were created based on that data. Completeness is measured by identifying null values or data elements in the database, and fields where data is missing entirely.
- Consistency roots out data redundancies and inconsistencies in values that are aggregations of each other. An example is a database in which the product model numbers used by the sales department don’t match the model numbers used by the production team.
- Freshness defines the currentness of the data at the present moment, which is related to but not synonymous with data timeliness, or the data’s relevance when applied to a specific task. For example, sales figures may be delayed from posting by an outdated roster of sales representatives. The sales data is accurate and timely for analysis, but it isn’t current.
Extrinsic metrics include relevance, reliability, timeliness, usability, and validity:
- Relevance ensures the data provides the necessary insight for the task, and is sufficient to meet all intended use cases. Irrelevance can be caused by redundancies, being out of date, or being incomplete.
- Reliability refers to how trustworthy stakeholders consider the data to be. For data to be considered true and credible, it must be verifiable in terms of its source, its quality, and any potential biases.
- Timeliness confirms that the data is up to date and available to be used for its intended purposes. Up-to-date information that never makes it to the decision-makers who need it is as useless as out-of-date information that gets to them right away.
- Usability determines how easily the data can be accessed and understood by the organization’s data consumers. The data must be clear and unambiguous, and it must be accessible using variations of request forms, wording, and approaches.
- Validity verifies that the data conforms to the company’s internal rules and data definitions. Various departments must agree on specific methods for creating, describing, and maintaining data to promote consistent and efficient business processes.
How to Improve Data Reliability: Examples and Challenges
Enhancing the reliability of your company’s data begins by identifying the most important use cases, such as sales forecasting, workforce planning, or devising effective marketing strategies. This lets you focus on the data that has the greatest organization-wide impact and provides common ground for all stakeholders. It also highlights the areas and applications in greatest need of more reliable data.
By adopting best practices for promoting data reliability, organizations realize benefits across the complete data stack: from data sources and extract and load tools, to cloud data warehouses and transformation tools.
- Adhere to data collection standards. This reduces variation in data and promotes consistency throughout the company.
- Train data collectors to focus on reliability. Make tools and techniques available to them that reduce the likelihood of human errors, and inform them of the costs associated with using unreliable data.
- Conduct regular audits. Data audits identify errors and inconsistencies in systems, and dig deeper to discover the causes of the problems and determine corrective actions.
- Test the reliability of your tools and instruments. Data collection instruments include surveys, questionnaires, and measuring tools. In addition to pilot testing the tools, you have to monitor the collection process for data completeness, accuracy, and consistency.
- Clean the data. Spot and remove any outliers in the data. Identify missing and inconsistent values and implement standard methods for achieving data completeness and consistency.
- Create a data dictionary. The dictionary serves as the central repository for data types, data relationships, and data meaning. It lets you track the source of the data, its format, and how it has been used. It also serves as a shared resource for all stakeholders.
- Make sure the data is reproducible. Carefully documenting your data collection practices allows you and others to reproduce your results. The methodologies used should be explained clearly, and all versions of data should be tracked accurately.
- Apply Data Governance policies. Make sure that the data consumers in the company understand your data policies and procedures relating to access controls, modifications, and updates to the change log.
- Keep your data backed up and recoverable. Prepare for the potential loss of critical data by testing your data recovery processes regularly.
Data Reliability Is Key for Building Trust in AI
The great promise of generative artificial intelligence (GenAI) depends on businesses and consumers overcoming their distrust of the technology. Data reliability can counteract the variability and inaccuracies that are inherent in large language model (LLM) machine learning systems. Applying data reliability principles to AI modeling addresses the implicit and explicit bias of AI-generated content.
Examples of data reliability applied to GenAI innovations include explainable AI (XAI) that enhances the transparency and understandability of the systems, and human-AI collaboration, which combines human intuition and experience with the computational efficiency of AI. Also under development are ethical AI frameworks that strive for fairness and equality in addition to accuracy and reliability.
Data is the fuel that powers modern business, but the value of that data declines precipitously as data consumers lose faith in its accuracy, integrity, and reliability. The best way to enhance the return your company realizes on its investments in data is to implement tools and processes that safeguard and enhance its value.